Machine Learning Approach to Antibiotic Discovery: Difference between revisions
(Created page with "<!-- Do not edit this line-->{{Curated}} ==Summary== Image:Stokes_et_al.png|thumb|300px|right|Graphical abstract from [https://www.ncbi.nlm.nih.gov/pubmed/32084340/ Stokes...") |
No edit summary |
||
Line 9: | Line 9: | ||
<br> | <br> | ||
Antibiotics are essential for the modern practice of medicine. However, the rise of antibiotic-resistant bacteria | Antibiotics are essential for the modern practice of medicine. However, the rise of antibiotic-resistant bacteria presents new challenges to this paradigm in medical treatment. Discovery and development of novel antibiotics is not meeting the pace of microbial resistance. The arms race against pathogenic bacteria has prompted significant research into new modes of antibiotic discovery. One rapidly expanding sector of this research uses a high throughput, machine learning approach to predict candidate antibiotics <i>in silico<i>. | ||
Line 19: | Line 19: | ||
==Traditional Discovery Methods== | ==Traditional Discovery Methods== | ||
One of the first experimental platforms for antibiotic discovery is known as the Waksman Platform. First implemented by the Rutgers microbiologist Selman Waksman, the Waksman Platform involved the screening of soil-borne actinomycetes on a field of overlaid bacteria<ref>[https://www.acs.org/content/acs/en/education/whatischemistry/landmarks/selmanwaksman.html]<ref>. Actinomycetes are bacteria which commonly produce antibiotics in their soil environment. The development of a zone of inhibition on the plate was the primary way candidate antibiotic-producing microbes were discovered. This technique was successfully used to discover several important antibiotics including streptomycin. While this platform was fundamental to finding many of the world’s antibiotics, it has certain limitations. First, there is a finite number of soil antibiotics. Approaches to sample more diverse soils and bacterial environments have been mostly unsuccessful in producing new classes of antibiotic<ref>[https://www.ncbi.nlm.nih.gov/pmc/articles/PMC4692294/ Valiquette, | |||
2015]<ref>. Second, the approach is not easily scaled up, meaning the process is slow and laborious. These reasons are some of many to prompt high throughput computational approaches to antibiotic discovery.<br> | |||
<br> | <br> | ||
==Methodology of Machine Learning in Antibiotic Discovery== | |||
A <ref>[https://www.sciencedirect.com/science/article/pii/S0092867420301021 paper]<ref> published in February of 2020 revealed their novel platform for the computational discovery of antibiotics. | |||
<br> | |||
==Advantages of Machine Learning in Antibiotic Discovery== | ==Advantages of Machine Learning in Antibiotic Discovery== | ||
New platforms for antibiotic discovery have overcome many of the challenges presented by the Waksman Platform. | |||
High throughput, less costly, quicker.<br> | High throughput, less costly, quicker.<br> | ||
<br> | <br> | ||
Line 37: | Line 42: | ||
==References== | ==References== | ||
<references /> | <references /> | ||
Valiquette, L., & Laupland, K. B. (2015). Digging for New Solutions. Canadian Journal of Infectious Diseases and Medical Microbiology, 26(6), 289–290. https://doi.org/10.1155/2015/971858 | |||
| |||
<br><br>Authored for BIOL 238 Microbiology, taught by [mailto:slonczewski@kenyon.edu Joan Slonczewski], 2018, [http://www.kenyon.edu/index.xml Kenyon College]. | <br><br>Authored for BIOL 238 Microbiology, taught by [mailto:slonczewski@kenyon.edu Joan Slonczewski], 2018, [http://www.kenyon.edu/index.xml Kenyon College]. |
Revision as of 15:38, 14 May 2020
Summary
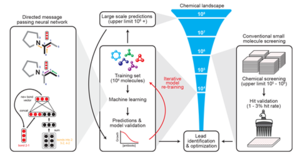
By Reed Crocker
Biology 238: Microbiology
Spring 2020
Antibiotics are essential for the modern practice of medicine. However, the rise of antibiotic-resistant bacteria presents new challenges to this paradigm in medical treatment. Discovery and development of novel antibiotics is not meeting the pace of microbial resistance. The arms race against pathogenic bacteria has prompted significant research into new modes of antibiotic discovery. One rapidly expanding sector of this research uses a high throughput, machine learning approach to predict candidate antibiotics in silico.
Sample citations: [1]
[2]
A citation code consists of a hyperlinked reference within "ref" begin and end codes.
To repeat the citation for other statements, the reference needs to have a names: "<ref name=aa>"
The repeated citation works like this, with a back slash.[1]
Traditional Discovery Methods
One of the first experimental platforms for antibiotic discovery is known as the Waksman Platform. First implemented by the Rutgers microbiologist Selman Waksman, the Waksman Platform involved the screening of soil-borne actinomycetes on a field of overlaid bacteria<ref>[1]<ref>. Actinomycetes are bacteria which commonly produce antibiotics in their soil environment. The development of a zone of inhibition on the plate was the primary way candidate antibiotic-producing microbes were discovered. This technique was successfully used to discover several important antibiotics including streptomycin. While this platform was fundamental to finding many of the world’s antibiotics, it has certain limitations. First, there is a finite number of soil antibiotics. Approaches to sample more diverse soils and bacterial environments have been mostly unsuccessful in producing new classes of antibiotic<ref>[https://www.ncbi.nlm.nih.gov/pmc/articles/PMC4692294/ Valiquette,
2015]<ref>. Second, the approach is not easily scaled up, meaning the process is slow and laborious. These reasons are some of many to prompt high throughput computational approaches to antibiotic discovery.
Methodology of Machine Learning in Antibiotic Discovery
A <ref>paper<ref> published in February of 2020 revealed their novel platform for the computational discovery of antibiotics.
Advantages of Machine Learning in Antibiotic Discovery
New platforms for antibiotic discovery have overcome many of the challenges presented by the Waksman Platform.
High throughput, less costly, quicker.
Future
Where this research might continue to.
Section 4
Conclusion
References
Valiquette, L., & Laupland, K. B. (2015). Digging for New Solutions. Canadian Journal of Infectious Diseases and Medical Microbiology, 26(6), 289–290. https://doi.org/10.1155/2015/971858
Authored for BIOL 238 Microbiology, taught by Joan Slonczewski, 2018, Kenyon College.